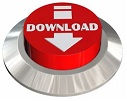

Testing strategies are central to achieve this. Once the number of infected people has successfully been brought sufficiently down, quick suppression of new waves of viral infections will be key.
#Net sensitivity for sequential testing how to#
The brief discusses what tests can be used for each goal, as well as practical implementation issues with testing strategies, including the opportunities and risks of using digital tools in this context.Ī key question behind any strategy to ease confinement restrictions and reopen economic activities is how to avoid a new spread of the SARS‑CoV‑2 virus that would necessitate further lockdowns. This brief discusses how testing strategies can be used to achieve three main goals: 1) suppressing the resurgence of local outbreaks 2) identifying people who have developed some form of immunity and can safely return to work and 3) gaining intelligence on the evolution of the epidemic, including on when a threshold for herd immunity has been reached. Crucially, quick suppression of infections requires testing more people to identify who is infected tracking them to make sure they do not spread the disease further and tracing with whom they have been in contact. If all confinement restrictions are lifted before a vaccine or effective treatments are developed without other measures to suppress new infections, the infection rate is expected to rebound rapidly. Finally, we make recommendations about how small the sample size should be relative to the population size to warrant use of the binomial model for prevalence estimation.This policy brief discusses the role of testing for COVID‑19 as part of any plan to lift confinement restrictions and prepare for a possible new wave of viral infections. Our findings indicate that the posterior standard deviations for prevalence (but not sensitivity and specificity) based on finite population sampling tend to be smaller than their counterparts for infinite population sampling. Using the developed methods, we analyse two real and one simulated data sets, and we compare 'hypergeometric' and 'binomial-based' inferences. We thus discuss joint (simultaneous and sequential) testing strategies and inference for them. Sequential versions of such strategies are often applied in order to reduce the cost of testing. Moreover, two tests are often applied simultaneously for the purpose of obtaining a 'joint' testing strategy that has either higher overall sensitivity or specificity than either of the two tests considered singly. In this paper, we develop statistical methods for evaluating diagnostic test accuracy and prevalence estimation based on finite sample data in the absence of a gold standard. child-care centre, village in Africa, or a cattle herd are sampled or if the sample size is large relative to population size.
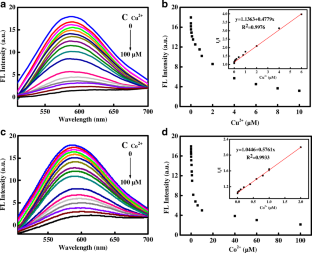
The binomial assumption is incorrect if all individuals in a population e.g.
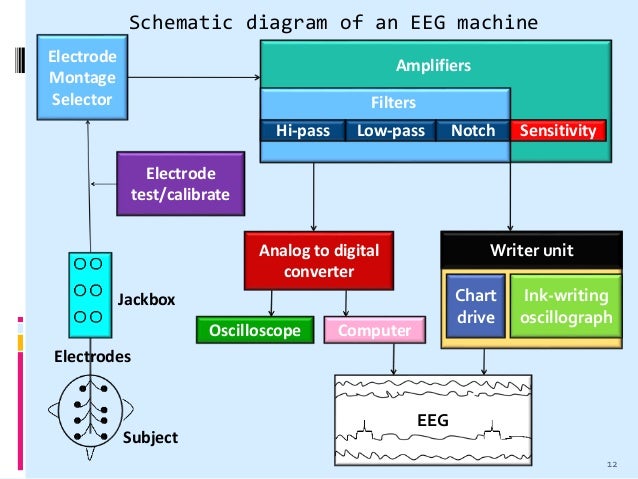
The two-test two-population model, originally formulated by Hui and Walter, for estimation of test accuracy and prevalence estimation assumes conditionally independent tests, constant accuracy across populations and binomial sampling.
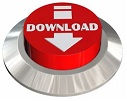